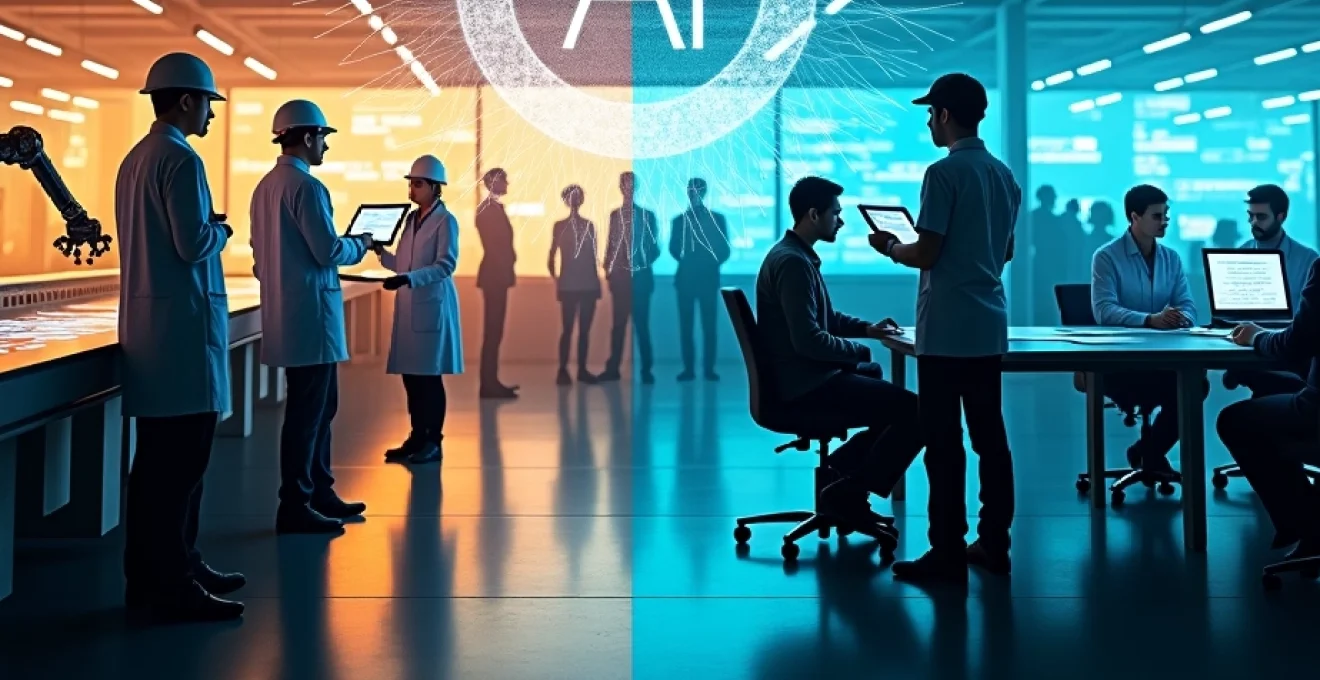
Artificial intelligence is rapidly reshaping the global job landscape in ways that were once confined to science fiction. This technological revolution is creating a profound economic shift comparable to the industrial revolution—simultaneously eliminating certain job categories while generating entirely new professional opportunities. According to the World Economic Forum, AI and automation could displace 85 million jobs by 2025, but may also create 97 million new roles. This transformation represents both a significant opportunity and a formidable challenge for workers, businesses, and governments worldwide.
As intelligent systems continue their march into diverse economic sectors, organizations find themselves at a critical juncture—needing to harness AI's efficiency benefits while managing workforce transitions thoughtfully. The technology's capacity to analyze vast datasets, learn from patterns, and execute complex tasks is transforming business models and operational strategies across industries. Understanding these changes is essential for professionals seeking to navigate an increasingly AI-influenced career landscape.
AI's impact on different economic sectors
The integration of artificial intelligence into various economic sectors is occurring at different rates and with varying implications. Each industry presents unique opportunities and challenges as intelligent automation reshapes traditional workflows. This technological adoption is driving productivity gains while simultaneously requiring workforces to adapt to new operational paradigms. Organizations that effectively implement AI solutions while managing talent transitions will likely emerge as leaders in their respective fields.
Manufacturing industry poised for automation boom
The manufacturing sector stands at the forefront of AI-driven transformation, with intelligent robotics and predictive maintenance systems revolutionizing production processes. Advanced computer vision technologies now enable quality control systems to detect minute defects invisible to the human eye, dramatically reducing error rates and waste. Meanwhile, collaborative robots—or "cobots"—are increasingly working alongside human operators, handling repetitive or physically demanding tasks while human workers focus on more complex operations requiring judgment and dexterity.
According to a McKinsey Global Institute report, manufacturing could see productivity increases of up to 20% through widespread AI implementation. This technological shift is particularly evident in automotive manufacturing, where AI-guided robots now handle everything from welding and painting to complex assembly tasks. However, this automation boom raises important questions about workforce transitions, as an estimated 20 million manufacturing jobs globally could be automated by 2030.
The sector is experiencing a significant skills gap as demand grows for workers who can program, maintain, and collaborate with intelligent systems. Factory workers increasingly need to develop hybrid skills that combine traditional manufacturing knowledge with technological proficiency. This transformation necessitates substantial investments in workforce retraining programs, particularly for mid-career professionals whose roles are most vulnerable to automation.
Healthcare transformed by intelligent diagnostic tools
The healthcare sector is witnessing a remarkable transformation as AI-powered diagnostic tools enhance clinical decision-making and improve patient outcomes. Machine learning algorithms can now analyze medical images with accuracy rivaling—and sometimes exceeding—human specialists, detecting subtle patterns invisible to the naked eye. These systems are particularly valuable in radiology, where AI can flag potential abnormalities in X-rays, MRIs, and CT scans, serving as a crucial "second opinion" for physicians.
Beyond imaging, AI applications in healthcare extend to predictive analytics for patient management, drug discovery acceleration, and personalized treatment recommendations. For instance, natural language processing
systems now analyze millions of medical records and research papers to identify optimal treatment protocols for specific patient profiles. These tools don't replace healthcare professionals but rather augment their capabilities, allowing them to focus more on patient interaction and complex decision-making.
The most significant impact of AI in healthcare may not be replacing clinicians but transforming how they work—shifting from reactive treatment to predictive and preventative care models that could dramatically improve patient outcomes while potentially reducing overall healthcare costs.
For healthcare professionals, this technological shift demands continuous learning and adaptation. Nurses and physicians increasingly need data literacy skills to effectively interpret AI-generated insights, while also maintaining the human touch that remains essential to quality care. Medical education programs are gradually incorporating AI-related training, recognizing that tomorrow's healthcare providers must be comfortable working alongside intelligent systems.
Financial services streamlined through predictive analytics
The financial services industry has emerged as an early adopter of artificial intelligence, with applications ranging from algorithmic trading to fraud detection and personalized banking. AI-powered risk assessment models now analyze thousands of variables to make lending decisions in seconds, while machine learning algorithms continuously monitor transaction patterns to identify potentially fraudulent activities with remarkable accuracy.
Investment management has been particularly transformed by quantitative analysis systems that can process vast amounts of market data to identify patterns and investment opportunities. These platforms don't merely execute trades faster than humans—they identify correlations and market signals that might escape human analysts entirely. Meanwhile, customer-facing AI applications like chatbots and virtual assistants have revolutionized retail banking, handling routine inquiries and transactions while freeing human staff for more complex customer needs.
For financial service professionals, this technological shift has created dual pressures: mastering new analytical tools while developing the interpersonal and advisory skills that remain distinctly human. Financial advisors increasingly function as interpreters of AI-generated insights, helping clients understand complex recommendations while providing emotional guidance during market volatility. This evolution requires a workforce with technical proficiency and heightened emotional intelligence—a combination not easily replicated by machines.
Emerging AI-Driven job roles & skills
As artificial intelligence transforms traditional work processes, it simultaneously generates demand for entirely new job categories that didn't exist a decade ago. These emerging roles often sit at the intersection of technology, domain expertise, and human factors—combining technical skills with contextual understanding and ethical considerations. Organizations across sectors are competing intensely for professionals who can bridge these domains, leading to premium compensation for individuals with the right skill combinations.
Data scientists in high demand across industries
Data scientists have emerged as the architects of the AI revolution, designing models that transform raw information into actionable business insights. These professionals combine advanced statistical knowledge with programming expertise and domain understanding to develop algorithms that can detect patterns, make predictions, and optimize processes. Their work forms the foundation for intelligent systems across sectors, from manufacturing optimization to personalized medicine and financial risk modeling.
The role requires proficiency in several technical domains, including:
- Advanced statistical analysis and mathematics
- Machine learning framework expertise (TensorFlow, PyTorch)
- Programming languages like Python and R
- Database management and data visualization
Beyond technical skills, effective data scientists must possess strong business acumen and communication abilities. They need to translate complex analytical findings into actionable recommendations for decision-makers who may lack technical backgrounds. This combination of technical depth and business savvy makes truly qualified data scientists relatively scarce, with demand significantly outpacing supply in most labor markets.
Organizations increasingly recognize that data science isn't merely a technical function but a strategic capability that can drive competitive advantage. As a result, experienced data scientists frequently command premium compensation packages and enjoy significant career mobility across industries. For professionals considering career transitions, data science represents one of the most promising pathways into the AI-driven economy, though it requires substantial investment in quantitative skills development.
Robotic engineers needed to develop intelligent machines
As physical automation accelerates across industries, robotic engineers have become essential for designing, implementing, and maintaining intelligent machines that can safely interact with humans and environments. These specialists bridge the gap between mechanical engineering, computer science, and artificial intelligence—creating systems that not only execute programmed tasks but can adapt to changing conditions through machine learning capabilities.
The field encompasses several specialized roles, including robotics software developers who create the control systems governing machine behavior, hardware engineers who design mechanical components, and integration specialists who ensure robotic systems work effectively within existing operational environments. Particularly valuable are engineers who understand both the technical aspects of robotics and the human factors involved when machines and people share workspaces.
Educational pathways into robotics engineering typically include degrees in mechanical engineering, electrical engineering, or computer science, often supplemented with specialized coursework in computer vision
, sensor systems, and machine learning. Practical experience with prototyping and testing is particularly valuable, as the field requires both theoretical knowledge and hands-on problem-solving abilities.
The demand for robotics expertise extends well beyond traditional manufacturing to emerging applications in healthcare (surgical robots), logistics (autonomous delivery vehicles), agriculture (harvesting systems), and consumer products (household robots). This cross-sector demand creates abundant opportunities for robotics specialists while contributing to competitive compensation levels that reflect their scarce and valuable skill sets.
AI ethicists crucial as technology advances
As artificial intelligence systems increasingly make consequential decisions affecting human lives, AI ethicists have emerged as essential guardians ensuring these technologies operate fairly, transparently, and in alignment with societal values. These professionals evaluate algorithms for potential biases, assess privacy implications, and develop frameworks for responsible AI deployment that balance innovation with ethical considerations.
The role typically combines technical understanding of AI systems with expertise in philosophy, law, and policy analysis. AI ethicists must comprehend how algorithms function technically while evaluating their broader societal impacts—a multidisciplinary skillset rarely found in traditional education programs. Their work includes conducting algorithmic impact assessments, developing ethical guidelines for AI applications, and creating governance frameworks that promote responsible innovation.
The most effective AI ethics practitioners operate as translators between technical teams, business leaders, and policy stakeholders—ensuring that ethical considerations are integrated throughout the development process rather than treated as an afterthought.
Organizations increasingly recognize that ethical AI deployment isn't merely about compliance but represents strategic risk management. Algorithms that produce biased outcomes or violate privacy expectations can create significant reputational damage and legal liability. Consequently, demand for AI ethicists has grown substantially as companies seek to navigate the complex intersection of technology capabilities and societal expectations.
For professionals interested in this emerging field, backgrounds in philosophy, social sciences, or law combined with technical literacy can provide entry points. Several universities now offer specialized programs in AI ethics and governance, reflecting the growing recognition that technological advancement must be balanced with ethical considerations to maintain public trust and maximize societal benefit.
Preparing the workforce for an AI-powered future
The accelerating integration of artificial intelligence across industries necessitates comprehensive workforce preparation strategies that address both immediate skill gaps and long-term career resilience. This preparation involves coordinated efforts across multiple stakeholders—educational institutions, employers, governments, and individuals themselves. The goal isn't merely adapting to current technologies but fostering adaptive capabilities that enable continuous learning as AI continues to evolve.
Educational institutions must adapt curricula rapidly
Traditional educational models—designed for relatively stable occupational categories and skills—face unprecedented pressure to evolve as AI transforms the knowledge and capabilities required for workplace success. Universities and vocational training programs are racing to update curricula that better prepare students for a landscape where technical skills may have increasingly shorter half-lives.
This adaptation extends beyond simply adding coding classes or data science programs. Forward-thinking institutions are fundamentally rethinking educational approaches to emphasize adaptability, critical thinking, and multidisciplinary perspectives. These qualities prove particularly valuable as AI increasingly handles routine cognitive tasks, shifting human work toward complex problem-solving and interpersonal domains that remain challenging to automate.
Several promising educational innovations have emerged:
- Modular micro-credentials that allow rapid skill acquisition without full degree commitments
- Industry-academic partnerships creating real-world learning environments with current technologies
- Problem-based learning approaches that develop transferable problem-solving capabilities
- Integrated humanities-technology programs that combine technical skills with ethical considerations
Educational institutions face significant challenges in this transition, including faculty expertise limitations, accreditation requirements designed for traditional programs, and the rapid pace of technological change that can render curriculum updates obsolete almost immediately. Institutions that successfully navigate these challenges will likely emerge as leaders in preparing talent for the AI-influenced economy, while those that maintain traditional approaches risk producing graduates with rapidly outdating skill sets.
Lifelong learning key to staying relevant
The accelerating pace of technological change has transformed learning from a time-limited academic phase to a continuous career requirement. Professionals across industries now recognize that skills obsolescence
represents a significant career risk in an AI-influenced economy, necessitating ongoing knowledge acquisition and capability development throughout their working lives.
This shift toward lifelong learning encompasses both formal education programs and self-directed skill development. Many mid-career professionals now regularly participate in targeted training programs, online courses, and professional certifications to maintain marketability as job requirements evolve. Organizations increasingly support these efforts through tuition assistance, dedicated learning time allocations, and internal skill development programs that help workers adapt to changing technological landscapes.
The most effective lifelong learning approaches balance technical skill acquisition with development of durable "human" capabilities that remain valuable regardless of technological evolution. While specific programming languages or analytical tools may change rapidly, skills like complex problem-solving, cross-functional collaboration, and adaptive thinking retain their value across technological transitions. This balanced approach creates career resilience by combining current technical relevance with enduring human capabilities.
For individuals navigating career development in an AI-influenced economy, strategic skill acquisition becomes essential. Rather than pursuing random credentials, effective learners analyze emerging market demands, identify personal skill gaps, and develop targeted learning plans that address specific capability deficits. They recognize that learning agility itself—the ability to quickly acquire new knowledge and skills—represents perhaps the most valuable meta-skill in a rapidly evolving workplace.
Governments businesses collaborating on reskilling initiatives
The scale of workforce transformation required by AI adoption exceeds what any single stakeholder can address independently. Recognizing this reality, governments and businesses are increasingly forming collaborative partnerships to develop and implement comprehensive reskilling programs. These initiatives aim to mitigate potential employment disruption while ensuring industries have access to the talent needed for continued growth and innovation in an AI-powered economy.
Public-private partnerships have emerged as particularly effective models for large-scale workforce development. In Singapore, the SkillsFuture program represents a comprehensive national upskilling initiative that combines government funding with industry-defined skill requirements. The program offers citizens learning credits for approved courses while working with businesses to identify emerging skill gaps and develop targeted training content. Similar initiatives have emerged across Europe, North America, and parts of Asia, reflecting a growing consensus that workforce adaptation requires coordinated action.
Ethical concerns surrounding widespread AI adoption
As artificial intelligence systems make increasingly consequential decisions affecting human livelihood and wellbeing, critical ethical questions have moved from theoretical discussions to immediate practical considerations. These ethical dimensions extend beyond technical performance to encompass fairness, transparency, privacy, and the fundamental question of how decision-making authority should be distributed between humans and machines.
Algorithmic bias represents one of the most pressing ethical challenges, as AI systems trained on historical data often replicate or even amplify existing societal inequities. This concern is particularly acute in employment contexts, where AI-powered recruiting tools have demonstrated biases against certain demographic groups. Research has shown that resume screening algorithms may disadvantage candidates from specific educational backgrounds or those with employment gaps—often disproportionately affecting women and minorities.
Privacy considerations present another critical ethical dimension, particularly as AI systems collect and analyze increasingly personal data to enhance performance. The tension between data utility and individual privacy rights creates complex tradeoffs that vary across cultural and regulatory contexts. Organizations deploying AI must navigate these considerations thoughtfully, recognizing that privacy violations can undermine public trust in intelligent technologies more broadly.
The question of decision-making transparency—often called the "black box
" problem—presents particular challenges for high-consequence applications. When AI systems make recommendations affecting employment, healthcare, or financial opportunities, individuals reasonably expect to understand the factors influencing these determinations. Yet many advanced AI architectures, particularly deep learning systems, operate in ways that make their decision processes difficult or impossible for humans to interpret fully.
Perhaps most fundamentally, AI adoption raises profound questions about human agency and dignity in increasingly automated environments. Societies must determine which decisions should remain exclusively human despite potential efficiency gains from automation. This includes establishing appropriate oversight mechanisms for high-consequence AI applications and ensuring that technological deployment ultimately serves human flourishing rather than merely maximizing operational efficiency.
Addressing these ethical concerns requires multidisciplinary approaches that include technical safeguards, organizational governance structures, and appropriate regulatory frameworks. Organizations at the forefront of responsible AI deployment are establishing internal ethics committees, conducting algorithmic impact assessments, and implementing continuous monitoring systems to detect and address unintended consequences as they emerge.
Humanity machine symbiosis the path forward
As artificial intelligence capabilities continue advancing, the most promising path forward appears not as competition between humans and machines but as thoughtfully designed collaboration that leverages the distinct strengths of each. This human-machine symbiosis represents a fundamental shift from viewing AI as a replacement technology to seeing it as a complementary tool that can enhance human capabilities while compensating for cognitive limitations.
The most effective AI implementations typically follow an augmentation strategy rather than a pure automation approach. Under this model, intelligent systems handle routine analytical tasks, data processing, and pattern recognition, while human workers provide contextual understanding, ethical judgment, and creative problem-solving. This division allows each to contribute their comparative advantages—machines excel at rapid processing of structured information, while humans navigate ambiguity and novel situations more effectively.
Several industries demonstrate this symbiotic approach in practice. In healthcare, AI diagnostic systems identify potential conditions from medical imaging, but physicians interpret these findings within the context of patient history and symptoms—combining algorithmic detection with human medical judgment. Similarly, in legal services, AI-powered contract analysis tools can review thousands of documents to identify relevant provisions, allowing attorneys to focus on strategic interpretation and client counseling rather than manual document review.
For this symbiotic relationship to flourish, both technological systems and workplace structures require thoughtful design. AI tools must be developed with human collaboration in mind, presenting information in ways that complement human cognitive processes rather than overwhelming them. Interface design becomes particularly critical, as poorly designed systems can create cognitive burden rather than reducing it, potentially negating productivity gains.
Equally important is cultural adaptation within organizations. Effective human-machine collaboration requires evolving beyond both technophobia and techno-optimism toward a nuanced understanding of how intelligent systems can enhance human work. This cultural shift often represents a greater implementation challenge than the technology itself, requiring leadership commitment, communication strategies, and recognition systems that reward collaborative innovation.