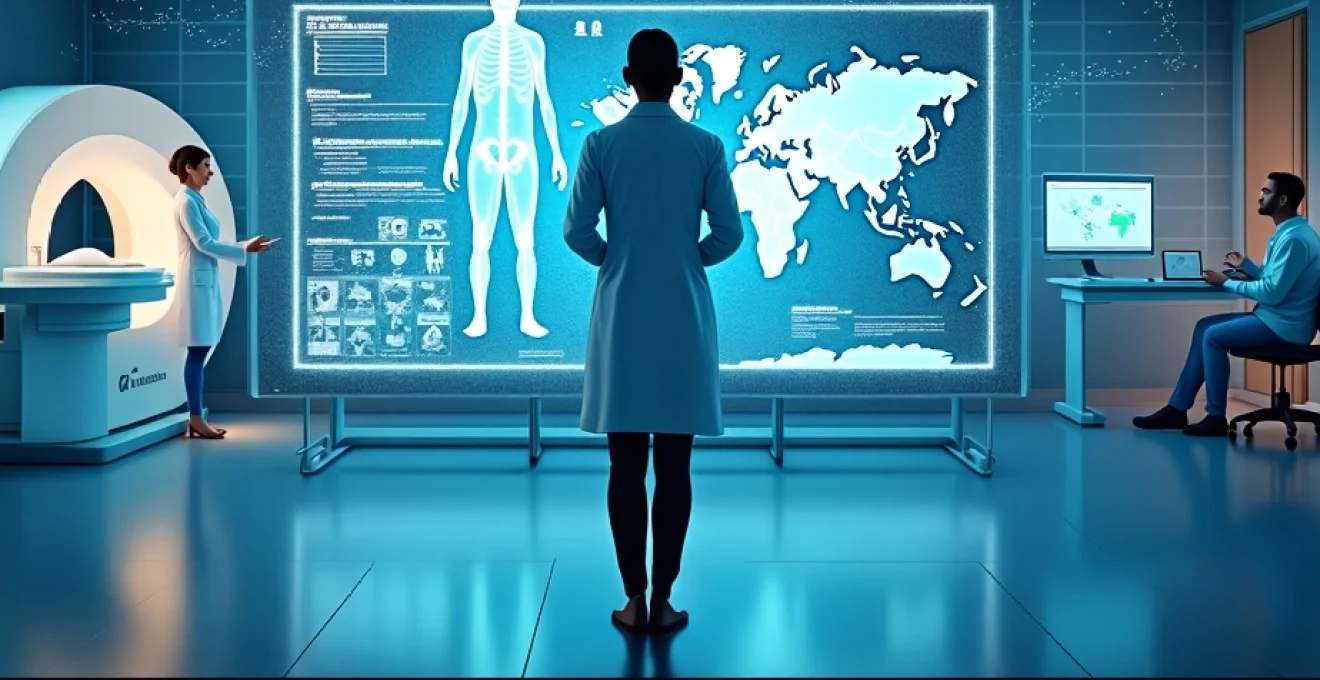
Artificial Intelligence (AI) is revolutionizing the healthcare industry, ushering in a new era of precision medicine, improved diagnostics, and enhanced patient care. From machine learning algorithms that assist in medical diagnosis to AI-powered robotic surgery systems, the applications of AI in healthcare are vast and rapidly evolving. This technological revolution is not only improving the efficiency of healthcare delivery but also enabling personalized treatment plans and accelerating drug discovery processes.
As AI continues to permeate various aspects of healthcare, it's crucial to understand its current applications and potential future impacts. Let's delve into the cutting-edge AI technologies that are transforming the medical landscape and explore how they're reshaping patient outcomes, clinical decision-making, and the overall healthcare ecosystem.
Machine learning algorithms in medical diagnosis
Machine learning algorithms are at the forefront of AI's impact on medical diagnosis. These sophisticated tools can analyze vast amounts of medical data, identify patterns, and make predictions with remarkable accuracy. By leveraging the power of AI, healthcare professionals can enhance their diagnostic capabilities, potentially catching diseases earlier and with greater precision than ever before.
Convolutional Neural Networks for medical image analysis
Convolutional Neural Networks (CNNs) have emerged as a game-changer in medical image analysis. These deep learning models excel at processing and interpreting visual data, making them invaluable in radiology and pathology. CNNs can rapidly analyze medical images such as X-rays, MRIs, and CT scans, detecting abnormalities that might be missed by the human eye.
For instance, CNNs have shown promising results in identifying early signs of lung cancer in chest X-rays, potentially speeding up diagnosis and improving patient outcomes. The ability of these networks to learn from millions of images allows them to recognize subtle patterns and features that indicate various medical conditions.
Natural Language Processing in electronic health records
Natural Language Processing (NLP) is transforming the way healthcare providers interact with Electronic Health Records (EHRs). By applying NLP techniques to the vast amount of unstructured data in medical records, AI systems can extract valuable insights, identify trends, and even predict potential health risks for patients.
NLP algorithms can sift through clinical notes, discharge summaries, and patient histories to highlight important information, flag potential drug interactions, and assist in clinical decision-making. This technology not only saves time for healthcare professionals but also reduces the risk of human error in data interpretation.
Random Forest models for predicting patient outcomes
Random Forest models, a type of ensemble learning algorithm, are proving to be highly effective in predicting patient outcomes. These models can analyze a wide range of patient data, including demographic information, medical history, and current health status, to forecast potential complications, readmission risks, and treatment responses.
By providing healthcare providers with these predictive insights, Random Forest models enable more proactive and personalized patient care. For example, they can help identify patients at high risk of developing sepsis, allowing for early intervention and potentially saving lives.
Support Vector Machines in cancer detection
Support Vector Machines (SVMs) are making significant strides in cancer detection and classification. These powerful algorithms can analyze complex datasets to distinguish between different types of cancer cells with high accuracy. SVMs are particularly useful in analyzing genetic markers and protein expressions associated with various cancers.
In breast cancer diagnosis, for instance, SVMs have been used to classify tumors as benign or malignant based on features extracted from mammography images. This application of AI not only improves diagnostic accuracy but also has the potential to reduce the need for invasive biopsies.
AI-powered robotic surgery systems
The integration of AI into robotic surgery systems is pushing the boundaries of surgical precision and minimally invasive procedures. These advanced systems combine the dexterity of robotic instruments with the decision-making capabilities of AI, offering surgeons enhanced control and accuracy during complex operations.
Da Vinci Surgical System: precision and minimally invasive procedures
The Da Vinci Surgical System is a pioneering example of AI-assisted robotic surgery. While not fully autonomous, it incorporates AI algorithms to enhance the surgeon's capabilities. The system translates the surgeon's hand movements into precise, scaled-down motions of miniature instruments inside the patient's body.
AI plays a crucial role in the Da Vinci system by providing real-time guidance, tremor filtering, and motion scaling. This allows for more precise incisions, reduced blood loss, and faster recovery times for patients. The system is particularly beneficial in procedures requiring high precision, such as prostate surgeries and gynecological operations.
ROSA brain: AI-assisted neurosurgical navigation
ROSA Brain is an AI-powered robotic assistant designed specifically for neurosurgical procedures. This system combines advanced imaging technologies with AI algorithms to provide surgeons with precise navigation and guidance during brain surgeries.
The AI component of ROSA Brain helps in planning the optimal trajectory for electrode placement in epilepsy surgeries or for tumor biopsies. It can analyze pre-operative imaging data to create detailed 3D models of the patient's brain, allowing surgeons to visualize and plan the least invasive approach to the target area.
Monarch platform: AI-guided bronchoscopy for lung cancer diagnosis
The Monarch Platform represents a significant advancement in the diagnosis of lung cancer. This AI-guided robotic bronchoscopy system enables physicians to navigate deep into the lungs with unprecedented precision, allowing for earlier and more accurate diagnosis of lung nodules.
AI algorithms in the Monarch Platform process real-time imaging data to guide the bronchoscope through the complex branching structure of the lungs. This technology enhances the ability to reach and biopsy small, peripheral lung nodules that were previously difficult to access, potentially leading to earlier detection and treatment of lung cancer.
AI in drug discovery and development
Artificial Intelligence is revolutionizing the pharmaceutical industry by accelerating drug discovery and development processes. AI technologies are enabling researchers to analyze vast amounts of biological data, predict molecular behaviors, and identify potential drug candidates more efficiently than ever before.
Deep learning models for molecular structure prediction
Deep learning models are making significant contributions to molecular structure prediction, a critical step in drug discovery. These AI systems can analyze large databases of known molecular structures to predict the 3D shapes of new compounds. This capability is crucial for understanding how potential drug molecules might interact with target proteins in the body.
For example, DeepMind's AlphaFold has made groundbreaking progress in protein structure prediction, a task that traditionally required months of laboratory work. By accurately predicting protein folding, AI is helping scientists identify new targets for drug development and design more effective medications.
Generative adversarial networks in compound design
Generative Adversarial Networks (GANs) are pushing the boundaries of de novo drug design. These AI systems consist of two neural networks that work against each other: one generates new molecular structures, while the other evaluates them. Through this process, GANs can create novel chemical compounds with desired properties.
Pharmaceutical companies are using GANs to explore vast chemical spaces and generate potential drug candidates that meet specific criteria, such as binding affinity to a target protein or predicted low toxicity. This approach significantly speeds up the initial stages of drug discovery by proposing promising molecules for further investigation.
Reinforcement learning for clinical trial optimization
Reinforcement learning, a type of machine learning where an AI agent learns to make decisions by interacting with its environment, is being applied to optimize clinical trial design and execution. This AI approach can help researchers determine the most effective dosing regimens, patient selection criteria, and trial durations.
By analyzing data from ongoing and past clinical trials, reinforcement learning algorithms can suggest adaptive trial designs that maximize the chances of success while minimizing risks to participants. This application of AI has the potential to reduce the time and cost associated with bringing new drugs to market, ultimately benefiting patients who need access to novel treatments.
AI-enhanced medical imaging technologies
Artificial Intelligence is transforming medical imaging, enhancing the quality of images, improving diagnostic accuracy, and increasing the efficiency of radiological workflows. AI algorithms are being integrated into various imaging modalities, from MRI and CT scans to X-rays and ultrasounds, revolutionizing how medical professionals interpret and utilize diagnostic images.
Deep learning in MRI image reconstruction
Deep learning techniques are significantly improving MRI image reconstruction, allowing for faster scan times and higher image quality. AI algorithms can reconstruct high-quality MRI images from undersampled data, reducing the time patients need to spend in the scanner while maintaining or even enhancing image resolution.
This application of AI not only improves patient comfort but also increases the throughput of MRI machines, potentially reducing waiting times for these critical diagnostic procedures. Moreover, AI-enhanced reconstruction can help in visualizing subtle anatomical details that might be crucial for accurate diagnosis.
AI-powered CT scan analysis for COVID-19 detection
The COVID-19 pandemic has accelerated the development and deployment of AI-powered CT scan analysis for detecting and assessing the severity of lung infections. AI algorithms can rapidly analyze chest CT scans to identify characteristic patterns of COVID-19 pneumonia, potentially assisting in early diagnosis and triage of patients.
These AI systems can quantify the extent of lung involvement and track changes over time, helping clinicians monitor disease progression and evaluate treatment efficacy. The speed and consistency of AI analysis can be particularly valuable in high-pressure situations where rapid decision-making is crucial.
Automated mammography screening using artificial intelligence
AI is making significant strides in mammography screening for breast cancer detection. Machine learning algorithms can analyze mammograms to identify suspicious areas that may indicate the presence of cancer, potentially catching abnormalities that human radiologists might miss.
These AI systems can serve as a "second reader," working alongside human radiologists to improve the accuracy of breast cancer screening. Some studies have shown that AI-assisted mammography can reduce false positives and false negatives, leading to more accurate diagnoses and potentially saving lives through earlier detection of breast cancer.
AI applications in personalized medicine
Personalized medicine, tailoring medical treatment to the individual characteristics of each patient, is one of the most promising frontiers in healthcare. Artificial Intelligence is playing a pivotal role in advancing this field, enabling more precise diagnostics, targeted therapies, and individualized treatment plans.
Genome sequencing and AI for tailored treatment plans
The combination of genome sequencing and AI is revolutionizing how we approach personalized medicine. AI algorithms can analyze an individual's genetic data to identify genetic variations that may influence their response to certain medications or their risk of developing specific diseases.
This genomic analysis allows healthcare providers to create tailored treatment plans that take into account a patient's unique genetic profile. For example, in oncology, AI can help determine which cancer treatments are most likely to be effective based on the genetic mutations present in a patient's tumor, potentially improving outcomes and reducing side effects.
Machine learning in pharmacogenomics
Pharmacogenomics, the study of how genes affect a person's response to drugs, is being significantly enhanced by machine learning techniques. AI algorithms can analyze large datasets that combine genetic information with drug response data to predict how individuals might react to specific medications.
This application of AI helps in identifying the most effective and safest drug options for individual patients, reducing the risk of adverse reactions and improving treatment efficacy. Machine learning models can also assist in dosage optimization, ensuring that patients receive the right amount of medication based on their genetic makeup and other individual factors.
AI-driven wearable devices for continuous health monitoring
AI-powered wearable devices are transforming continuous health monitoring, providing real-time data that can be used to personalize healthcare interventions. These devices, ranging from smartwatches to specialized medical sensors, can track various health parameters such as heart rate, blood pressure, glucose levels, and physical activity.
The AI algorithms embedded in these devices can analyze the continuous stream of data to detect patterns, predict potential health issues, and alert both the user and healthcare providers to any concerning changes. For example, AI-driven wearables can help in managing chronic conditions like diabetes by providing personalized recommendations for medication, diet, and exercise based on real-time glucose monitoring.
As AI continues to advance, its applications in healthcare are likely to expand even further, promising a future where medical care is increasingly precise, personalized, and proactive. The integration of AI across various aspects of healthcare, from diagnosis and treatment to drug discovery and patient monitoring, is not just improving existing processes but fundamentally reshaping how we approach health and wellness.